---
author: Stephen J. Mildenhall
categories:
- notes
date: '2025-01-13'
description: 'Tail and body behavior of stable distributions.'
draft: false
image: img/banner.png
title: Stable Distributions
code-line-numbers: true
code-copy: true
code-overflow: wrap
code-fold: true
code-tools: true
---
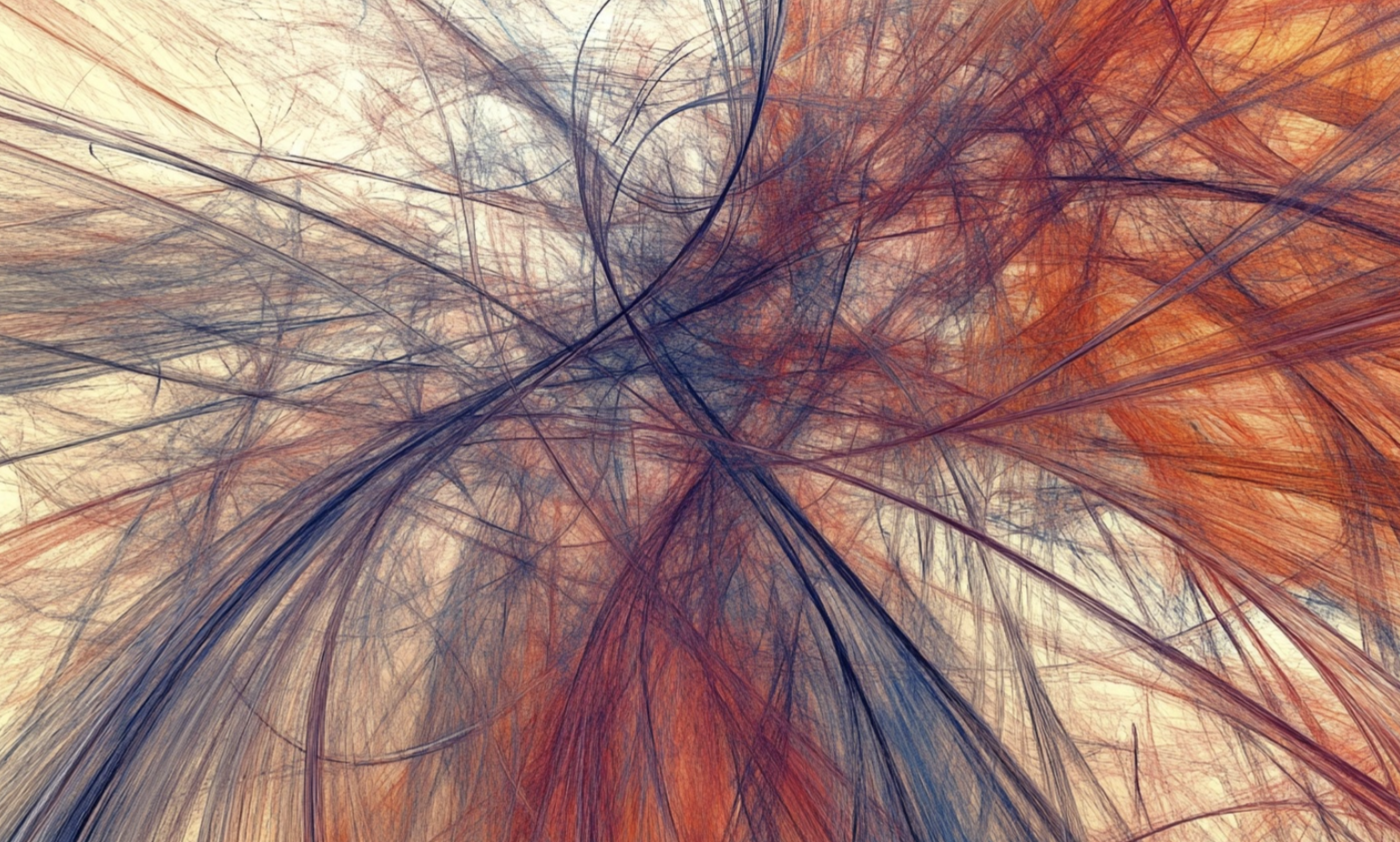 {width=33%}
Stable distributions are defined by tail index $\alpha\in (0, 2]$, skewness $\beta\in[ -1,1 ] $, scale $\gamma>0$ and location $\delta$. The tail index and skewness are shape parameters. The properties of $\mathrm{St}(\alpha, \beta, 1, 0)=\mathrm{St}(\alpha, \beta)$ depend critically on $\alpha$. There are two aspects: body behavior which is determined by small jumps, and tail behavior determined by large. @fig-one shows the options.
```{python}
#| echo: true
#| label: fig-one
#| fig-cap: "Behavior of stable distributions. Small jumps determine the body and support. Large jumps determine tails."
import matplotlib.pyplot as plt
import numpy as np
def anno(ax, txt, xy, xytext, opts, arrow_color= 'black' ):
return ax.annotate(
txt, # Text to display
xy= xy, # Point the arrow points to
xytext= xytext, # Offset label position (x, y)
arrowprops= dict (arrowstyle= "->" , color= arrow_color, lw= .5 ,
connectionstyle= "arc3,rad=0" ), # Arrow style
** opts )
def make_dia():
# Define x values
x = np.hstack((np.linspace(1 / 1000 , 1 , 999 , endpoint= False ), np.linspace(1 , 10 , 1000 )))
# Define the functions
f1 = 1 / x
f2 = 1 / x** 2
f3 = 1 / x** 3
# Create the figure and axis
fig, ax = plt.subplots(figsize= (5 , 5 ))
ax.axvline(1 , ymin= 0 , ymax= .25 , lw= .5 , c= 'k' , alpha= 0.5 , ls= '--' )
# Plot the functions
ax.plot(x, f1, label= r"$1/x$" , color= "blue" )
ax.plot(x, f2, label= r"$1/x^2$" , color= "red" )
ax.plot(x, f3, label= r"$1/x^3$" , color= "black" )
# Shade areas
ax.fill_between(x, f2, f1, where= (f2 < f1), color= "blue" , alpha= 0.2 , label= "A1" )
ax.fill_between(x, f3, f2, where= (x >= 1 ), color= "red" , alpha= 0.2 , label= "A2" )
ax.fill_between(x, f3, 0 , where= (x >= 1 ), color= "yellow" , alpha= 0.2 , label= r"A3" )
# hatch='//',
ax.fill_between(x, f2, 0 , where= (x < 1 ), #hatch='\\\\\\',
lw= .5 , ec= 'blue' , fc= 'green' , alpha= 0.05 , label= "SA4" )
ax.fill_between(x, f2, f3, where= (x < 1 ), hatch= '////' , lw= .5 , ec= 'red' , color= 'red' , alpha= 0.2 , label= r"A5" )
# Annotate regions
opts = {'ha' : 'center' , 'va' : 'center' , 'fontsize' : 8 }
ax.text(0.5 , 0.65 , 'Finite small \n jump length \n subordinators \n with half-line \n support possible \n (green)' , ** opts)
opts = {'ha' : 'left' , 'va' : 'bottom' , 'fontsize' : 8 }
anno(ax, 'No mean \n (blue)' , (2.5 , 1 / 2.75 - 0.125 ), (2.75 , 0.45 ), opts)
sh = - 0.1
anno(ax, 'Finite mean \n no variance \n (orange)' , (2 + sh, (1 / 4 + 1 / 8 )/ 2 + 0.0 ), (2.25 + sh, .7 ), opts)
opts['ha' ] = 'right'
anno(ax, 'Finite mean \n and variance \n (yellow)' , (1.5 , 1 / (1.5 ** 3 ) - 0.2 ), (2 , 1 ), opts)
opts['ha' ] = 'left'
eps = 0.015
xpad = .6
anno(ax, '$1/x$, $ \\ alpha=0$, e.g., Levy' , (1 / 3.5 - eps, 3.5 ), (1 / 3.5 + xpad, 3.5 ), opts, arrow_color= 'blue' )
anno(ax, '$1/x^2$, $ \\ alpha=1$, Cauchy' , (1 / 3.25 ** 0.5 - eps, 3.25 ),
(1 / 3.25 ** 0.5 + xpad, 3.25 ), opts, arrow_color= 'red' )
anno(ax, '$1/x^3$, $ \\ alpha=2$' , (1 / 3.0 ** (1 / 3 )- eps, 3.0 ), (1 / 3.0 ** (1 / 3 ) + xpad, 3.0 ), opts, arrow_color= 'black' )
opts['ha' ] = 'center'
x = 3.4
y = .7
anno(ax, '$1/x$' , (x, 1 / x- eps), (x, y), opts, arrow_color= 'blue' )
x = 3.625
y = .6
anno(ax, '$1/x^2$' , (x, 1 / x** 2 - eps), (x, y), opts, arrow_color= 'red' )
x = 3.85
y = .5
anno(ax, '$1/x^3$' , (x, 1 / x** 3 - eps), (x, y), opts, arrow_color= 'black' )
y = 2.
x = (y** (- 1 / 3 ) + y**- .5 ) / 2
opts['ha' ] = 'left'
opts['va' ] = 'center'
anno(ax, 'Infinite activity \n support $(- \\ infty, \\ infty)$ \n (orange hatched)' , (x- eps, y), (x + .4 , y), opts)
# Label axes
ax.set_xlabel(r"Jump size, $x$" , fontsize= 9 )
ax.set_ylabel(r"Jump frequency, $\nu(dx)$" , fontsize= 10 )
# Add legend
# ax.legend(loc='upper right', fontsize=9)
ax.set (ylim= [- 0.05 , 4 ], xlim= [- 0.05 , 4 ])
ax.set_xticks([0 , .5 , 1 , 2.5 , 4 ])
ax.set_xticklabels(['0' , 'small \n jumps' , '1' , 'large jumps \n control tail' , '$ \\ cdots$' ])
ax.set_yticks([0 , 1 , 4 ])
ax.set_yticklabels(['0' , '1' , '$ \\ infty$' ])
ax.set_xticks([1.5 , 2 , 3 , 3.5 ], minor= True )
ax.set_yticks([2 , 3 , 4 ], minor= True )
make_dia()
```